一.Encoder与Decoder的意义
在单纯使用RNN进行generation时其生成的东西非常pool,以生成文本为例,RNN只能通过学习后输出与输入语言风格类似的句子,但我们无法对句子的内容进行控制,因此无法实现模拟对话等功能。
因此可以采用Encoder-Decoder模型进行训练。以文本翻译为例,Encoder指将输入的句子先通过一次RNN,从而生成一个vector,再将此vector输入另一个用于输出翻译结果的RNN,此RNN即称作Decoder。在Decoder中每一个time均将Encoder中生成的vector作为输入,从而避免后续节点忘记训练的内容。
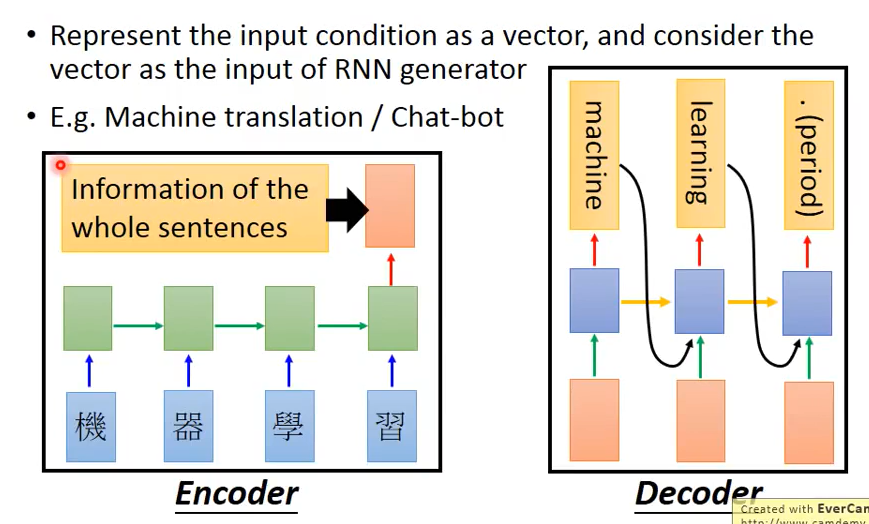
二.attention model的意义
attention model能够使得decoder中输入的vector每个time都不一样,此操作的意义在于:1.Encoder中的输入可能非常复杂,无法用一个vector来描述 2.可以让Decoder专注于它所需要的信息,如encoder输入机器学习,则decoder的第一个time仅希望考虑机器两个字而不是一整个句子,因此通过这个方式machine可以学的更好。
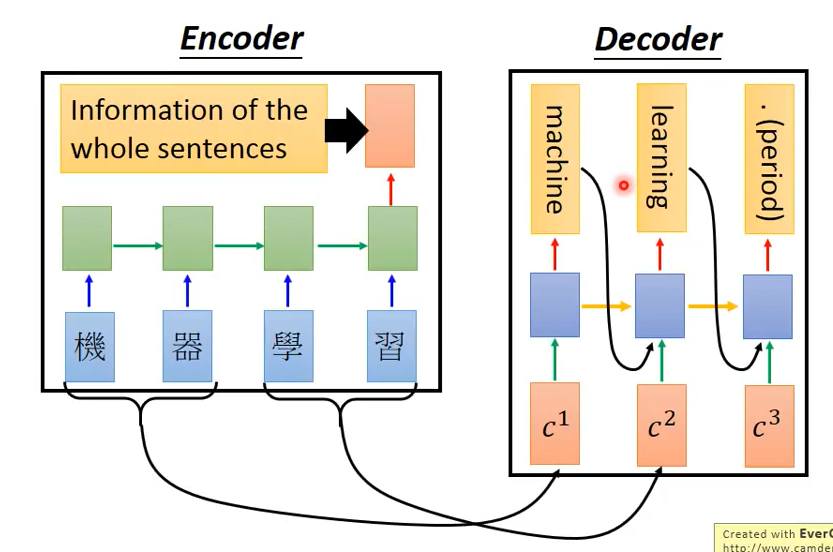
三.attention-based model建立步骤
以机器翻译为例
1.定义初始参数$z^0$,将$h^i$(RNN的hidden layer的output)与$z^0$通过match函数计算得到$\alpha ^i_0$,其中0表示此时计算time为0,match函数可以自行设计,常见的有cosine similarity of z and h等
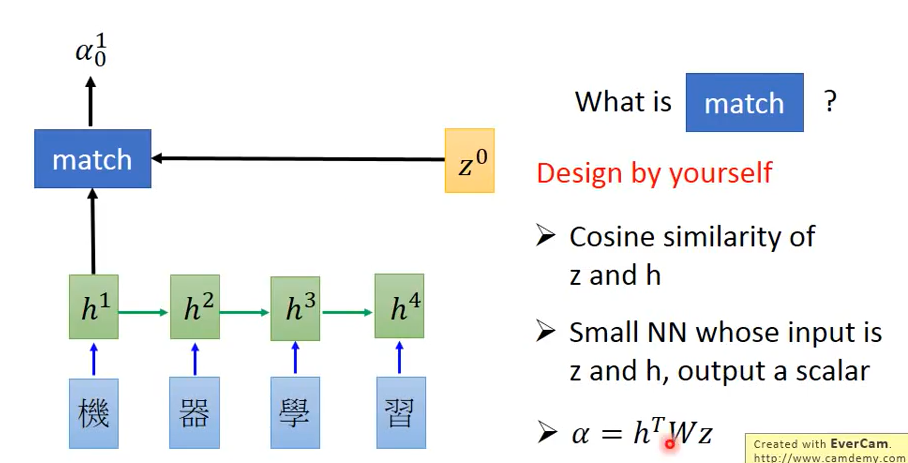
2.将$\alpha ^i_0$经过softmax层生成$\hat{\alpha}^i_0$,再利用公式$c^0= \sum_{i=1}^n \hat{\alpha}^i_0 h^i $得到$c^0$。则$c^0$的值将受到$\hat{\alpha}^i_0$的影响,从而实现不同的time专注于不同的信息
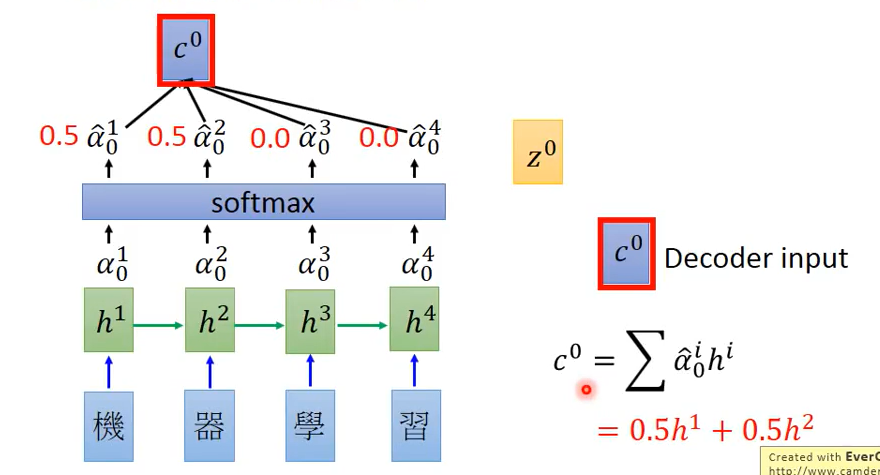
3.将得到的$c^0$输入Decoder中,则得到第一个输出machine,其中训练得到的$z^1$为RNN中的hidden layer output,可再将其作为参数放入Encoder进行训练,从而得到$c^1$。通过重复上述步骤从而完成训练。
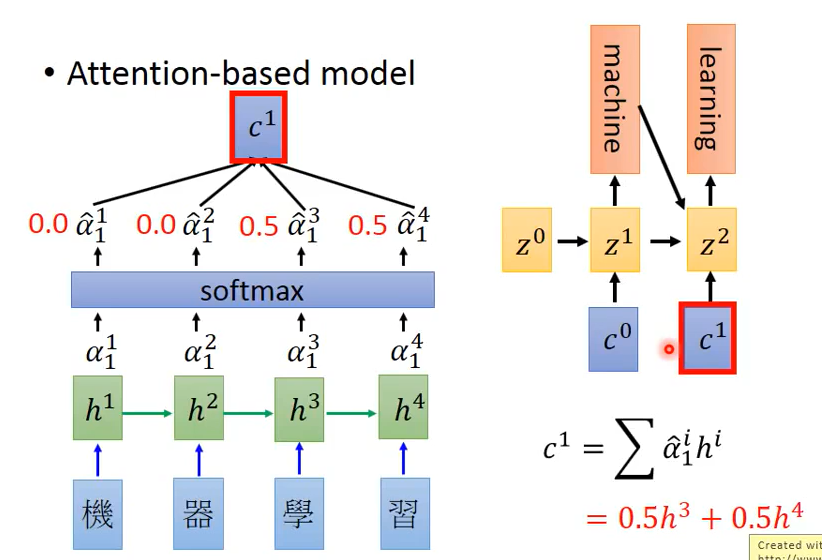
四.attention-based model在Image Caption Generation的应用
基本思路:利用CNN对一张图像进行训练,将模型中作flatten之前的filter output取出,利用attention-based model逐步生成word,则能够得到能够描述图片的一段句子
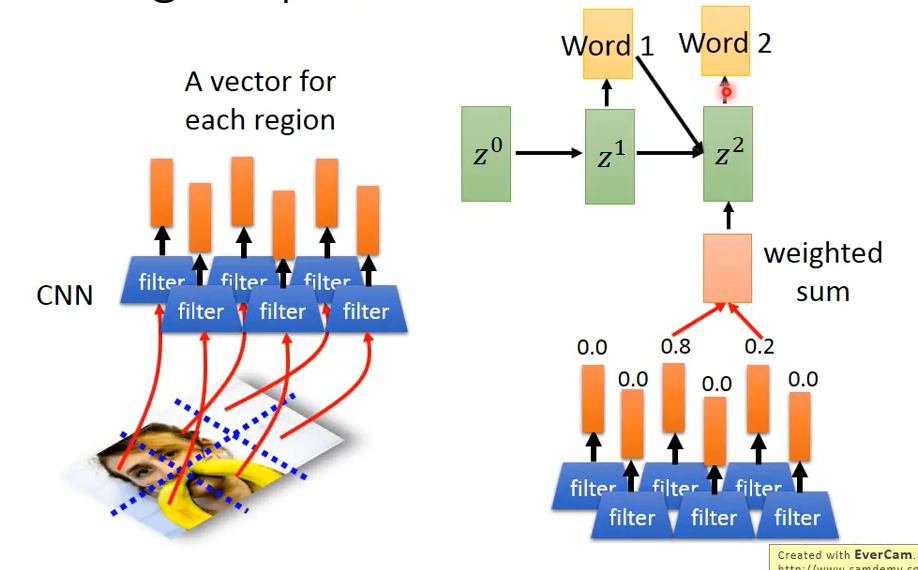
Comment